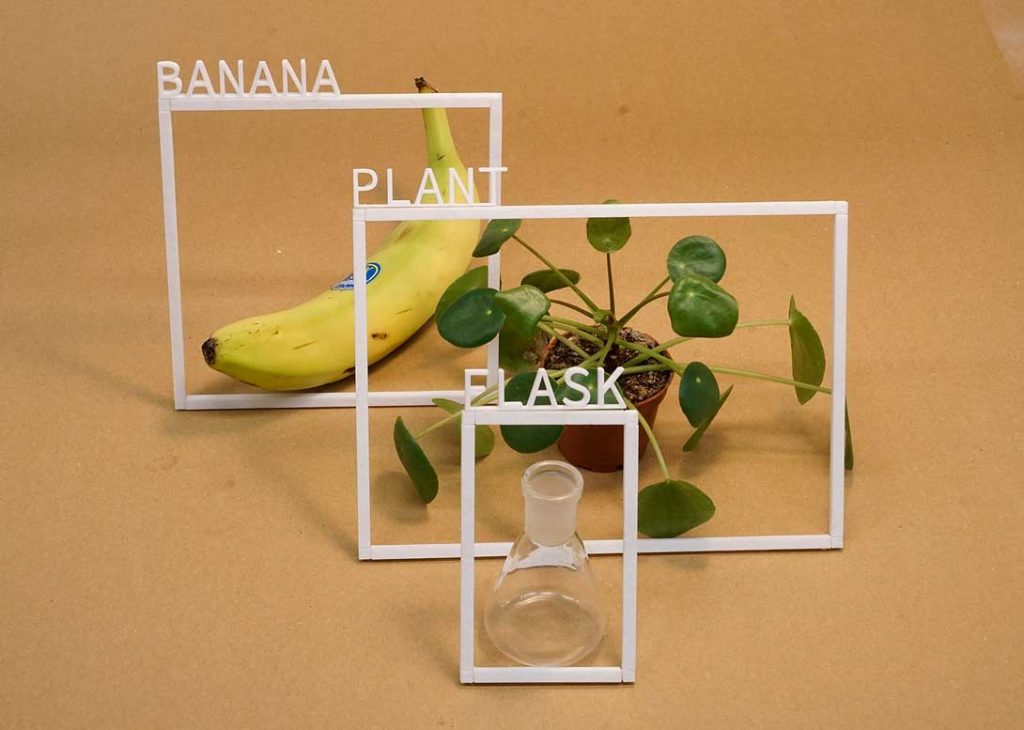
Vikas Agarwal, an expert in Artificial Intelligence, Machine Learning, and Cloud Computing, writes a special column for Deccan Mirror explaining the role of synthetic data in AI.
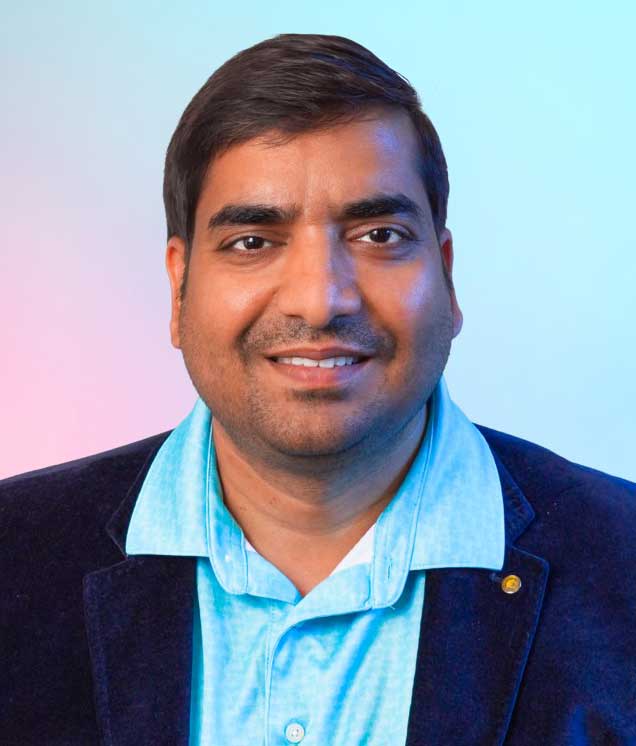
Data is everything in the digital age. It opens the Pandora’s box of umpteen numbers of options for utilizing it on multiple platforms for multiple purposes. Enter the scene, AI and ML, the importance of data has grown ever since, more and more important. The demographic challenges, privacy concerns, access to awareness on usage of data, and limitations of diversity have led to a revolutionary alternative called synthetic data.
Synthetic data refers to artificially generated information that mimics real-world datasets. Created using algorithms, simulations, or generative models like GANs (Generative Adversarial Networks), this data serves as a substitute for actual records while maintaining similar statistical properties. This is the new norm of using synthetic data everywhere in the fields that needed data inputs and processing.
The AI systems that needed huge and high-quality datasets for training models have given way to synthetic data. The limited access to sensitive information and possible chances of biases, which were a result of reflection of societal prejudices, and the availability of rare data, like medical case information, have often become impossible. The importance of synthetic data generated from artificially generated simulations and scenarios has become a part of modern data training models. Their tailored datasets generated from synthetic data are customizable, scalable, and privacy-preserving.
To help it explain the practicality of it, we can list a few here. When a patient’s privacy becomes questionable and restricted, the best way to improve diagnostics and treatment procedures, the synthetic data becomes the best tool to achieve it without compromising privacy. The data of autonomous vehicles comes up with the same kind of road scenarios to generate proper algorithms for auto-driving mode. Simulating customer behavior data also allows companies to refine their personalization methods without needing to access consumer data. The app is used to detect frauds in synthetic data.
The relevance of synthetic data again comes to a question of whether the generated data is how close to the real world. It is arguable how much bias they can really avoid, as they were programmed by algorithms generated by human beings. Google’s Gemini comes close to raising questions about the trustworthiness of synthetic data.
Putting aside the arguments, the emergence of artificially generated data sets is surely going to improve the quality of AI engines and thus improve the quality of the study and understanding about the models they are used in. Advances in generative AI tools, such as OpenAI’s DALL·E and DeepMind’s AlphaFold-inspired techniques, are expected to enhance the quality and dependability of synthetic datasets. Synthetic data, with all its package of arguments and pros, is going to unlock many new possibilities and bridge the gap between innovation and privacy. Let’s await more magic opening up from the advent of synthetic data.